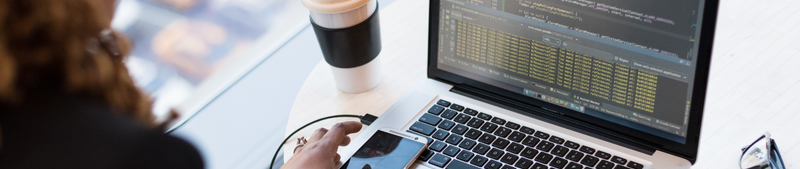
All Data is NOT Created Equal: How DonorSearch and MLR Data Leaves RFM in the Dust
On the scale of epic mishaps, crashing a spacecraft worth $327 million into a planet has to rank pretty high up there. That’s exactly what happened to Nasa’s Mars Climate Orbiter in 1999. The accident occurred because half the team was measuring in inches and the other half in centimeters. In other words, no amount of precision could provide the right answers.
Many nonprofits face a similar challenge. This is due to an overreliance on RFM data. Although they’re not risking an interplanetary disaster, nonprofits are harming their chances of achieving their worthy causes. At a time in which corporations are courting philanthropic customers with benevolent mission statements, it’s more critical than ever for nonprofits to level the data playing field with their for-profit peers.
Before advancing solutions, let’s discuss why many corporations dumped RFM more than a decade ago. The metric, which stands for Recency, Frequency, Monetary Value, is often used to rank particular donors as compared to the overall community. Corporations applied the same approach with their customers until they realized the metric didn’t sufficiently answer this question: “Who should we be talking to more?”
A Harvard Business Review article published twenty years ago described RFM’s downfall. It’s truer now than ever. In the piece titled, “The Mismanagement of Customer Loyalty,” authors Werner J. Reinartz and V. Kumar lay out the importance organizations of all types place on loyalty. Then they build a case that RFM is a poor measurement to rely on, mostly because it’s slow to update. Example: the article describes a company who has a customer with a very high RFM score. This favorable rating was due to the customer making a large initial purchase.
The problem? They hadn’t made another purchase in years.
This scenario is familiar ground for every non-profit exec struggling to keep donor data updated. But there is a bigger issue with RFM. It often doesn’t provide accurate info because it isn’t measuring the right things. Case in point: the Harvard Business Review authors found RFM scores are based on clumsy metrics. These don’t reflect the true underlying customer relationship amongst the corporations they studied.
The same thing haunts nonprofits. Just consider this description of the problems with RFM, then reflect on how it might impact your organization:
We were able to predict how profitable and how loyal any particular customer would be with 30% more accuracy than we obtained using traditional methods like RFM. That kind of misinformation carries a high price. Our mail-order company, for instance, was sending mailings to people it should have ignored, ignoring people it should have been cultivating, and sending the wrong material to people.
It’s time for nonprofits to realize what corporations knew 20 years ago. RFM scores don’t provide the accuracy needed for fundraising success.
Enter DonorSearch. We developed an RFM alternative providing accurate and actionable data by harnessing the power of AI. Even better, we make it available for free to every screening client. Now, let’s talk about the DonorSearch MLR score and why it might be RFM’s “boosted cousin.” Our metric, MLR for “Most Likely to Respond,” identifies individuals most likely to respond to an appeal for charitable support from your organization.
The fundamental difference between RFM and MLR, and what drives all the advantages of our approach, is that MLR uses AI for a more complex analysis of constantly updated data. Meanwhile, RFM remains a simple mathematical score one might use Excel to calculate. The application of AI algorithms and machine learning to a nonprofit’s donor community helps locate high potential donors that might never pop up on the RFM radar.
Clearly, AI-powered MLR scoring possess serious advantages over legacy approaches. First, the scores are more accurate. That’s because MLR uses 3-5 years of detailed gift transactions in its calculations, not summary or aggregate data. Next, MLR includes many more variables in its calculations—especially data points critical to your organization’s success.
As DonorSearch Senior Vice President Nathan Chappell explains, “Your community of donors and potential donors can’t be boiled down to just three data points. Our approach enables organizations to consider many at once, creating a more holistic view. Nonprofits are often shocked by deeply engaged prospects that score highly on MLR yet are nowhere to be found in an RFM analysis. This is thanks to the dozens of data points we build into our tool.”
As the name implies, RFM is based on total gifts, amount of the last gift, and data on the last gift. Now, compare this to MLR. It contains more than 90 data points, including email history, event participation, volunteering, and external factors like big data demographics and detailed relationship mapping. Combined with machine learning that’s always fine-tuning the algorithm and updating scores, the net result is an accurate picture of your donor base. It “lives and breathes” instead of lagging behind actual conditions on the ground.
For nonprofits seeking to do good in the face of skyrocketing costs, nothing is more vital than speaking to the best prospects as often as possible. DonorSearch Executive Vice President and Co-Owner Sarah TeDesco explains, “Nonprofits must up their game in a challenging economy. Their missions remain just as important—if not more so—but the cost of achieving results continues to climb. The answer? Build engagement, strengthen ties with existing donors, and find new prospects you haven’t focused on before. DonorSearch built the solution in the MLR score, and we’re ready to help you reach unprecedented fundraising success.”
If you’re ready to leave RFM in the dust, please contact DonorSearch for a demo today. And if you’re an experienced non-profit professional interested in helping nonprofits achieve their goals, consider a career with DonorSearch.